Understanding Data Analysis Courses
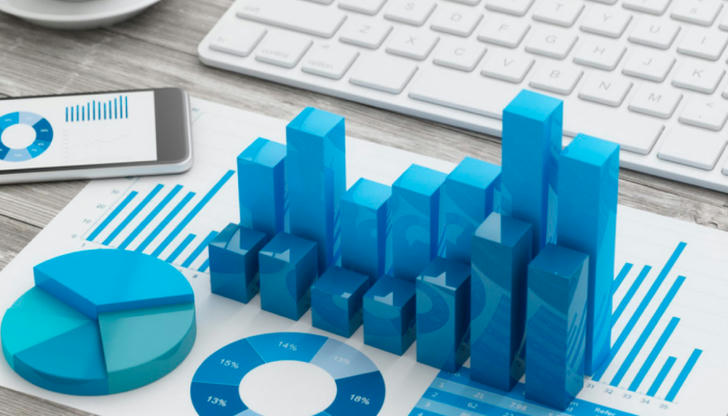
In today’s rapidly evolving world, data is the new gold. From healthcare to finance, marketing to education, data plays a crucial role in shaping decisions and strategies. However, raw data alone is not valuable; it is through data analysis that organizations can gain insights, make informed decisions, and achieve operational excellence. Data analysis is a multifaceted field that involves collecting, cleaning, processing, and interpreting data to uncover meaningful patterns and trends. To succeed in this data-driven world, professionals and organizations alike need to equip themselves with data analysis skills. One of the most effective ways to acquire these skills is through formal education in the form of data analysis courses. These courses offer foundational knowledge, hands-on experience, and the technical tools needed to navigate the complex landscape of data. In this article, we will explore what data analysis courses encompass, the skills they impart, and why they are critical in the current job market. Additionally, we will delve into the different types of data analysis courses available and what learners can expect from them.
The Importance of Data Analysis
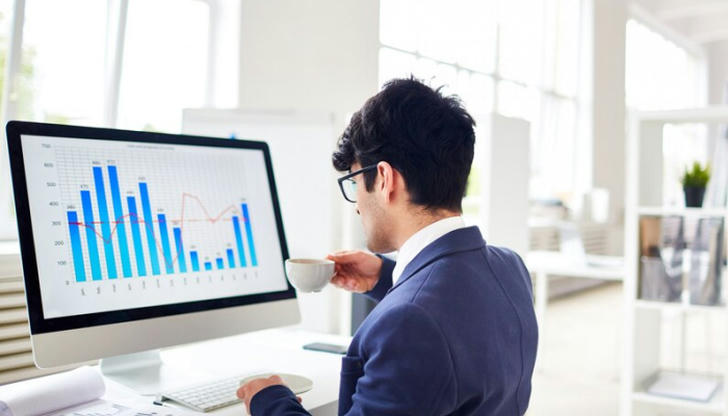
Before delving into data analysis courses, it is important to understand why data analysis is critical today. In the past, decisions in business, healthcare, government, and various sectors were often based on intuition or historical trends. However, with the massive amounts of data being generated every second—whether through social media, online transactions, or sensor data—the need for data-driven decisions has become more apparent. Data analysis involves examining large sets of data to draw conclusions about that information. It helps organizations: 1.Make Informed Decisions: Data analysis allows companies to base decisions on facts and insights rather than assumptions. Whether it’s predicting customer behavior, identifying market trends, or understanding financial health, data analysis can help businesses make decisions that are backed by evidence. 2.Optimize Operations: By analyzing operational data, organizations can identify inefficiencies, streamline processes, and improve productivity. For example, businesses can use data analysis to optimize inventory management, reduce waste, and forecast demand. 3.Enhance Customer Insights: Understanding customer preferences, buying patterns, and feedback is crucial for businesses aiming to offer personalized services. Data analysis helps companies understand customer behaviors, allowing them to tailor products and services that meet customer needs. 4.Predict Future Trends: Data analysis can uncover trends that provide valuable insights into future behaviors. Through predictive modeling and forecasting, businesses and organizations can anticipate changes in the market, prepare for demand fluctuations, and adapt their strategies accordingly. 5.Given its increasing importance, data analysis has become a core competence for professionals in various industries. As a result, data analysis courses have gained immense popularity, helping individuals acquire the skills necessary to analyze and interpret data effectively.
What Do Data Analysis Courses Entail?
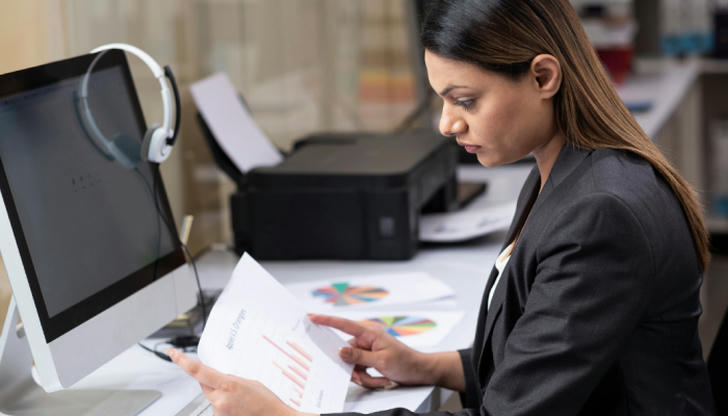
Data analysis courses are designed to introduce learners to the principles, techniques, and tools used in the field of data analysis. These courses typically cover a broad range of topics, equipping students with a comprehensive understanding of the data analysis process. Below are some of the key components typically found in data analysis courses.
- Data Collection and Data Cleaning The first step in any data analysis process is to gather the relevant data. Data analysis courses teach learners the importance of collecting accurate and reliable data from various sources. They also emphasize the necessity of data cleaning—the process of removing errors, inconsistencies, or missing values from the data set. Data cleaning is critical because inaccurate or incomplete data can lead to flawed conclusions. Courses will teach techniques such as: •Handling Missing Data: Learners are trained to identify and handle missing data through methods like imputation or deletion, ensuring the integrity of the dataset. •Removing Outliers: Inaccurate or extreme data points that deviate significantly from the rest of the data can distort analysis. Courses guide students on how to detect and manage outliers. •Data Transformation: Data often needs to be transformed or normalized before analysis. Data analysis courses teach learners techniques such as standardization, categorization, and encoding, allowing data to be analyzed more effectively.
- Exploratory Data Analysis (EDA) Exploratory Data Analysis is a crucial phase in the data analysis process. It involves visually inspecting the data to identify trends, patterns, and anomalies before conducting more advanced statistical analyses. EDA is critical because it helps analysts get a sense of the data, formulate hypotheses, and decide on the appropriate analytical methods. Courses typically teach learners to use visualization tools such as: •Histograms and Box Plots to understand the distribution of data. •Scatter Plots to examine relationships between variables. •Heatmaps to visualize correlations among different data points. In addition to visualization, students will also be introduced to basic statistical methods used in EDA, such as measures of central tendency (mean, median, mode) and measures of variability (variance, standard deviation).
- Statistical Analysis Statistical analysis is at the heart of data analysis, allowing professionals to draw meaningful conclusions from data. Data analysis courses provide students with a solid foundation in both descriptive statistics (summarizing data) and inferential statistics (making predictions or generalizations about a population based on a sample). Students typically learn to: •Apply Hypothesis Testing: Learners are introduced to techniques like t-tests, chi-square tests, and ANOVA to compare groups and test the validity of hypotheses. •Correlation and Regression Analysis: These techniques help students understand relationships between variables. Regression models, such as linear regression, allow learners to predict future trends or outcomes based on historical data. •Probability Distributions: Courses also introduce the concept of probability distributions, which model uncertainties and randomness in data. Examples include normal distributions, binomial distributions, and Poisson distributions. By the end of this section of the course, learners will be able to conduct robust statistical analyses and make data-driven conclusions about trends, patterns, and relationships in the data.
- Machine Learning and Predictive Analytics As the field of data analysis continues to evolve, machine learning has become an integral part of the discipline. Predictive analytics and machine learning techniques are now frequently used to analyze vast amounts of data and generate insights that inform decision-making. Data analysis courses often cover: •Supervised Learning: This includes methods such as linear regression, logistic regression, decision trees, and support vector machines. Learners will also be exposed to the concept of training and testing datasets, evaluating models using metrics like accuracy and F1-score. •Unsupervised Learning: Courses introduce techniques such as k-means clustering, hierarchical clustering, and principal component analysis (PCA), which are used to uncover hidden patterns or structures in the data without predefined labels. •Model Evaluation: Data analysis courses teach students to evaluate the performance of machine learning models using various metrics (e.g., confusion matrix, ROC curve, precision, recall) to ensure the reliability of their predictions. Students may also learn how to deploy machine learning models to make real-time predictions or develop dashboards that provide actionable insights.
- Data Visualization Data visualization is an essential skill in modern data analysis, as it helps translate complex data sets into understandable insights. Effective visualizations can communicate trends, patterns, and relationships more clearly than raw numbers or tables. Courses provide learners with the skills needed to: •Create Interactive Dashboards: Students learn how to use tools like Tableau, Power BI, or Google Data Studio to create dynamic visualizations that enable users to explore data and gain insights interactively. •Design Meaningful Charts: Courses focus on best practices for designing charts and graphs, including choosing the right chart type (e.g., bar chart, pie chart, line graph) and making visualizations that are both informative and aesthetically pleasing. A key aspect of data visualization is ensuring that visuals accurately represent the data without misleading the viewer. Students learn the ethical implications of data visualization and how to avoid common pitfalls like cherry-picking data or manipulating visuals to exaggerate trends.
Types of Data Analysis Courses
Data analysis courses are offered in various formats and can be categorized based on the depth of knowledge required, the type of tools taught, and the learning platform. Here are some common types of data analysis courses:
- Online Data Analysis Courses Online platforms like Coursera, edX, Udacity, and LinkedIn Learning offer a wide range of data analysis courses, catering to both beginners and experienced analysts. These courses are often self-paced and cover a broad spectrum of topics, from the basics of data analysis to advanced machine learning techniques. Many of these courses also provide certification upon completion, which can be valuable for career advancement.
- University Degree Programs For those looking for a more structured and comprehensive education, university degree programs in data science, statistics, or business analytics offer in-depth courses in data analysis. These programs typically offer a combination of theoretical knowledge and practical skills, preparing students for careers in data-driven roles such as data analysts, data scientists, and business intelligence professionals.
- Bootcamps and Workshops For individuals seeking a more immersive learning experience, data analysis bootcamps and workshops are intensive, short-term programs designed to teach hands-on data analysis skills quickly. These bootcamps usually focus on practical applications, offering students the chance to work on real-world projects. Bootcamps like General Assembly, DataCamp, and Le Wagon are popular among those looking to transition into data careers.
Challenges and Opportunities in Modern Early Childhood Education
While modern early childhood education has made tremendous progress, there are still several challenges that need to be addressed. Some of the key challenges include:
- Access and Equity Despite the benefits of high-quality early childhood education, access remains a significant issue, especially for children from low-income or marginalized communities. Ensuring that all children, regardless of their socioeconomic background, have access to quality early education is essential for closing achievement gaps and promoting social equity.
- Teacher Training and Professional Development The success of modern early childhood education depends heavily on the quality of the teachers. Educators need to be well-trained in child development, teaching strategies, and classroom management. Professional development programs are essential to help teachers stay current with best practices and evolving educational trends.
- Balancing Tradition with Innovation Modern ECE programs must strike a balance between traditional educational methods and the integration of new technology and teaching strategies. While innovation is important, it is equally important to maintain the value of hands-on learning, play, and face-to-face interactions that have long been recognized as essential for young children's development.
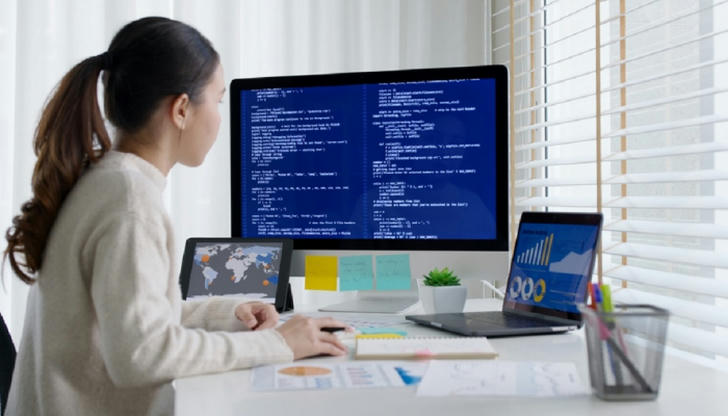
Conclusion
Data analysis courses are crucial for anyone looking to enter or advance in the field of data science or analytics. By covering everything from data collection and cleaning to machine learning and data visualization, these courses provide the knowledge and skills required to transform raw data into actionable insights. With the increasing importance of data in decision-making across industries, acquiring data analysis skills has become a valuable investment for professionals and organizations alike. Whether you’re a beginner seeking to learn the basics or an experienced professional looking to refine your skills, there are data analysis courses available to meet your needs. By gaining proficiency in data analysis, individuals not only enhance their career prospects but also contribute to the larger trend of data-driven decision-making that is shaping the future of business, technology, and society.